S u m m a r y
|
We present a knowledge-driven segmentation process with a nonlinear
statistical shape prior. It extends our work on statistical
shape knowledge in Mumford-Shah based segmentation.
|
We present a variational integration of nonlinear shape statistics
into a Mumford-Shah based segmentation process. The nonlinear
statistics are derived from a set of training silhouettes by a novel
method of density estimation which can be considered as an extension
of kernel PCA to a stochastic framework.
The idea is to assume that the training data forms a Gaussian
distribution after a nonlinear mapping to a potentially
higher-dimensional feature space. Due to the strong nonlinearity, the
corresponding density estimate in the original space is highly
non-Gaussian. It can capture essentially arbitrary data
distributions (e.g. multiple clusters, ring- or banana-shaped
manifolds).
Applications of the nonlinear shape statistics in segmentation and
tracking of 2D and 3D objects demonstrate that the segmentation
process can incorporate knowledge on a large variety of complex
real-world shapes. It makes the segmentation process robust against
misleading information due to noise, clutter and occlusion.
The main contributions of our approach are:
-
A novel statistical shape model on the basis of Mercer kernels.
-
A probabilistic framework for kernel PCA.
-
A variational integration as a nonlinear statistical shape prior into
a Mumford-Shah based segmentation process.
-
Numerical results which demonstrate the capacity of the nonlinear
shape prior to encode a large variety of fairly different real-world
shapes, such as the silhouettes of various 3D objects seen from
different views.
|
D e m o n s t r a t i o n s
|
Here you can find demonstrations (mpeg movies) of our method.
All movies were downsampled to lower resolution to reduce the amount
of data.
Click on the images to watch the evolution of the segmenting contour.
|
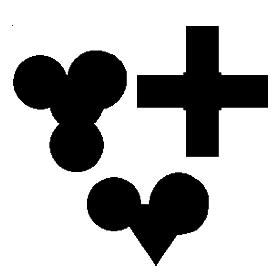 | The basic principle: The
nonlinear shape prior is capable of simultaneously encoding a number
of fairly different shapes. In this example the three objects shown
on the left were used as a training set for generating the nonlinear
shape prior. Adding this prior to the segmentation process permits
to segment partially occluded (or in other ways corrupted) versions of
these objects. The prior is invariant with respect to similarity
transformations, this means that the given objects can be segmented
even if they are translated, scaled or rotated. In this example
the nonlinear prior is added to the segmentation process as soon as
the purely data-driven segmentation process is stationary.
|
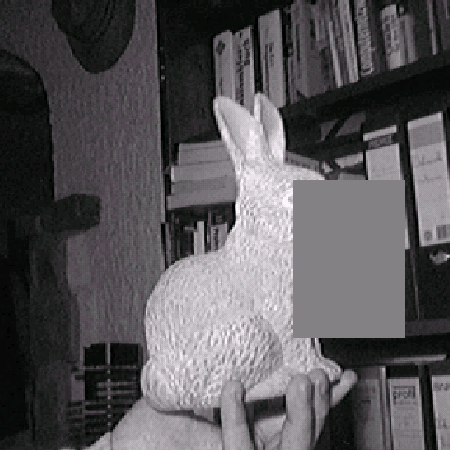 | Tracking: The nonlinear
shape prior is capable of encoding a 3D object in terms of various 2D
views. Due to the shape prior, background clutter is ignored and
the silhouette of the object is reconstructed in areas of
occlusion. The bottom right window of the movie shows a 2D
projection of the evolving contour (in white) which demonstrates how
the nonlinear prior forces the contour towards the domain of familiar
shapes (black boxes). |
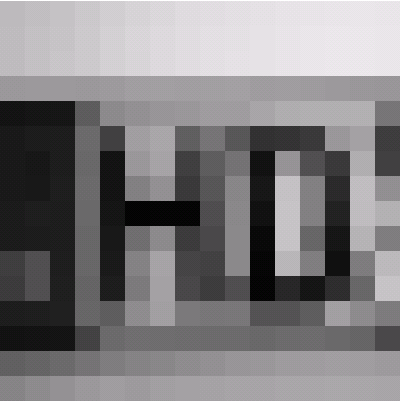 |
Application:
We encoded a set of letters into the nonlinear prior. Added to the
segmentation process, the nonlinear prior strongly improves the
segmentation of smoothed low-resolution images of these letters. In
the movie, we demonstrate this for segmenting two of the trained
letters (with the same nonlinear prior). Upon introduction of the
prior, we change the colour of the contour (from red to purple) for
better visibility. We do not claim that this is the most
competitive method for optical character recognition. The results
merely demonstrate the wide applicablity of the nonlinear prior for
knowledge-driven segmentation. |
|
R e l a t e d   P u b l i c a t i o n s
|
Articles in Journals
-
"Shape Statistics in Kernel Space for Variational Image Segmentation"
[D. Cremers, T. Kohlberger & C. Schnörr] , Pattern
Recognition, to appear, 2002.
Conference Articles
|