S u m m a r y
|
We propose to extend the Mumford-Shah functional for image
segmentation by a statistical shape energy which favors the
formation of ``familiar'' contours. We recently extended this
method to incorporate a nonlinear
statistical shape prior.
|
We present a modification of the Mumford-Shah functional and its
cartoon limit which facilitates the incorporation of statistical prior
on the shape of the segmenting contour. By minimizing a single energy
functional, we obtain a segmentation process which maximizes both the
grey value homogeneity in the separated regions and the similarity of
the contour with respect to a set of training shapes. The statistical
prior is automatically generated on the basis of a set of binary
training images.
We propose a closed-form, parameter-free solution for incorporating
invariance with respect to similarity transformations into the
variational framework. As a consequence the evolving contour is
restricted to a subspace of familiar shapes while being entirely free
to translate, rotate and scale.
We show segmentation results on artificial and real-world images with
and without prior shape information. In the case of noise, occlusion
or strongly cluttered background the shape prior significantly
improves segmentation. Finally we compare our results to those
obtained by a level-set implementation of geodesic active contours.
The main contributions of our approach are:
-
The diffusion snake: A modification of the
Mumford-Shah functional for spline contours.
-
A variational framework for segmentation which combines region-based
low-level cues with a higher-level statistical shape prior.
-
A closed-form, parameter-free solution for the incorporation of
similarity invariance into the shape energy.
-
Numerous demonstrations of the capacity of the shape prior to
compensate for missing or misleading information due to noise, clutter
or occlusion.
|
D e m o n s t r a t i o n s
|
Here you can find demonstrations of our method.
The examples
on the left show segmentation results for the diffusion snake without
any statistical shape prior. In contrast, the examples on the right
show the corresponding segmentation process with a statistical shape
prior, generated automatically from a set of binary training
images.
Click on the images to watch the evolution of the
segmenting contour. |
Segmentation without Statistical Shape Prior |
Explanation |
Segmentation with a Statistical Shape Prior |
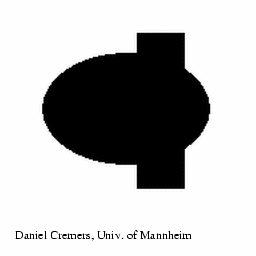 |
The basic principle: The statistical shape prior restricts the evolving contour to a subspace of familiar shapes. The resulting knowledge-driven segmentation process becomes robust against noise, clutter and occlusion. |
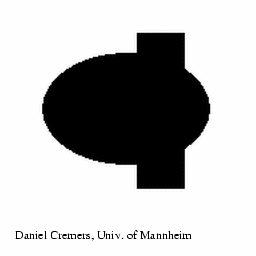 |
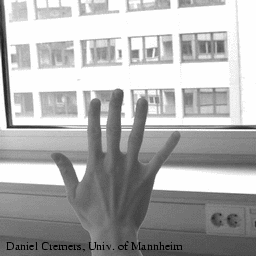 |
Cluttered
Background: Due to the statistical shape prior, the background clutter is ignored. |
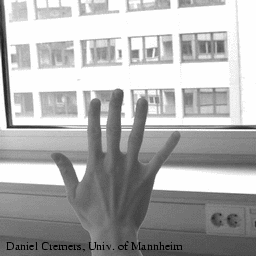 |
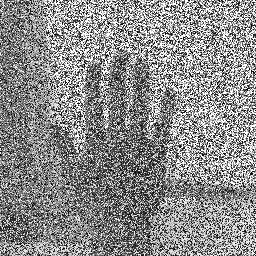 |
Noisy Input Image: Segmentation on an image with 75% random noise. The statistical shape prior compensates for missing information due to the noise. |
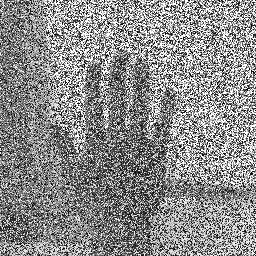 |
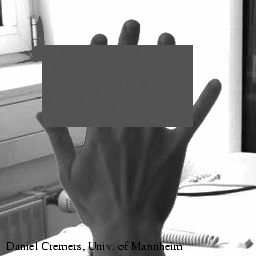 |
Partially Occluded Input
Image: Due to the statistical shape prior, the silhouette
of the object of interest is reconstructed in parts where it is
occluded. In contrast, the corresponding segmentation process
without shape prior is not able to produce the desired segmentation
(even if optimally initialized - see movie on the left). |
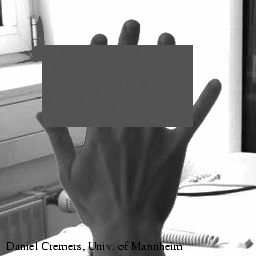 |
|
R e l a t e d   P u b l i c a t i o n s
|
Articles in Journals
-
"Diffusion Snakes: Introducing Statistical Shape Knowledge into the
Mumford-Shah Functional"
[D. Cremers, F. Tischhäuser, J. Weickert & C. Schnörr] , International Journal of Computer Vision, to appear,
2002.
Conference Articles
-
Diffusion-Snakes Combining
Statistical Shape Knowledge and Image Information in a Variational Framework
[D. Cremers, C. Schnörr & J. Weickert] , IEEE Workshop on Variational and Levelset Methods, Vancouver, July 2001, best student paper award.
-
Learning
Translation Invariant Shape Knowledge for Steering Diffusion Snakes
[D. Cremers, C. Schnörr, J. Weickert & C. Schellewald] , 3rd Workshop on Dynamic Perception, University of Ulm, Nov. 2000
-
Diffusion
Snakes using Statistical Shape Knowledge
[D. Cremers, C. Schnörr, J. Weickert & C. Schellewald] ,
Algebraic frames for the perception-action cycle, Kiel Sept. 2000,
Springer LNCS, Vol.
1888
|