Synthetic Biology
Next-generation tools for gene circuit design
Synthetic Biology aims at reprogramming cells with custom functionalities. Microbial cell factories, in particular, promise to tap into the metabolism of a host to produce chemicals like therapeutic drugs and other high value compounds. Some of our current work in this space:
-
Deep learning for strain design. We are exploring the power and limits of machine learning methods for predicting protein expression. To this end we use large genotype-phenotype screens to train and test a variety of ML methods, including deep neural networks and transfer learning.
-
Gene circuits for metabolic engineering. We produce computational methods to design gene circuits with the ability to control metabolic function in a prescribed manner. In this area we use control theory, nonlinear dynamics and optimization theory to find robust circuit architectures that optimize production of high-value chemicals.
-
Host-circuit interactions. We are interested in building predictive models that describe the interplay of synthetic gene circuits and the physiology of their cellular host. We make extensive use of mechanistic models for bacterial growth that describe the dynamic allocation of resources between native and foreign processes.
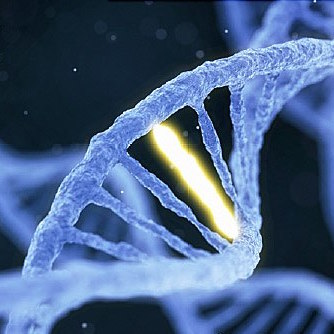